Table Of Content
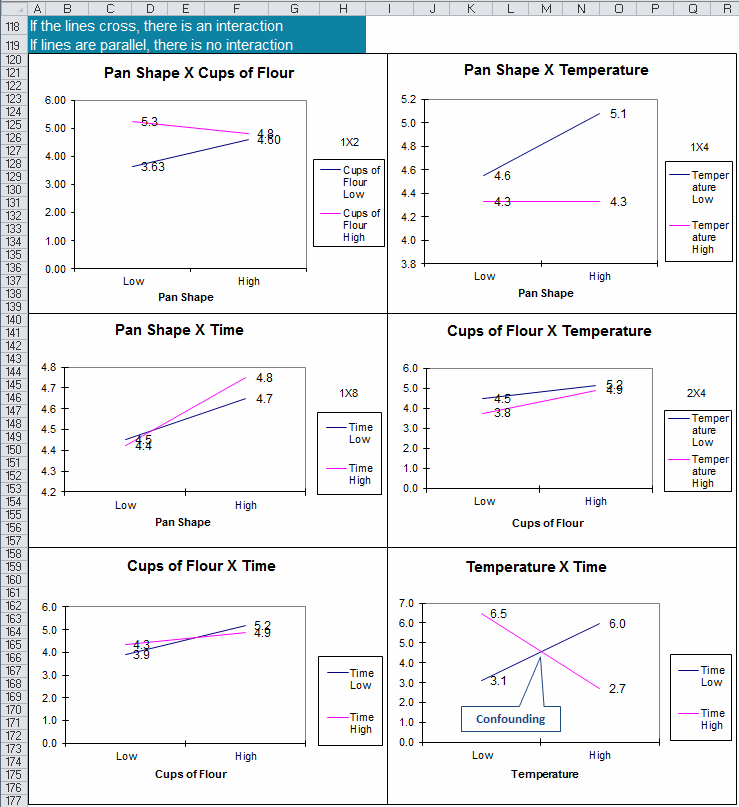
The genetic payload will need to be at least partially rewritten, for instance. Getting a eukaryote to stably express the gene or genes of interest can be more difficult than with prokaryotic lines. In general, the more complex organisms become, the greater the stochasticity in behavior and systems tend to get noisier. Maintaining relative stability means considering a greater number of factors and inputs compared with simpler organisms. DoE is a useful tool for determining specific factors affecting defect levels in a product, which may be used to improve the design of the product.
Statistics Knowledge Portal
You can also determine the objective, whether arriving at a solution, increasing the quality to a benchmark, or reducing the process time. In many cases, it’s less important to get strictly the highest amount of something you possibly can than to get at least some minimum amount (2 g/l yield, for example). Similarly, it’s likely that there will be a maximum amount of certain impurity you can tolerate from the process. Optimization allows us to impose constraints on responses to achieve things like this.
main types of DOE designs, explained
Once the data for a particular factor or level is collected, proceed to the next level of treatment combination. Replication of the experiments is important to confirm the statistical significance of the data. Fractional factorial designs assume that while there may be many effects, only a few are important. In other words, interactions between 2 factors—are more common and more influential than higher order effects (typically, interactions between more than 3 factors). A fractional factorial design takes a rational sample of the experimental landscape to provide a balanced, structured design that generates explanatory and predictive models. Full factorial designs are often most appropriate when screening has identified a few important factors to optimize, or when using liquid handling automation affording an increase in throughput.

Process Improvement
By knowing this you can design a product or process that meets or exceeds quality requirements and satisfies customer needs. Factorial Design explores every possible combination of factors and levels within a single experiment, providing comprehensive data on the main effects and interactions between factors. This design is invaluable for experiments where understanding the synergistic effects of multiple factors is crucial for drawing accurate conclusions. There are multiple approaches for determining the set of design points (unique combinations of the settings of the independent variables) to be used in the experiment. The research integrates Computational Fluid Dynamics (CFD) with optimization analysis theory. Various typical geometric structures of desert highway roadbed were modeled using the Design Exploration module in CFD.
What is the Scientific Method?
For example, we can estimate what we call a linear model, or an interaction model, or a quadratic model. So the selected experimental plan will support a specific type of model. The important thing here is that when we start to evaluate the result, we will obtain very valuable information about the direction in which to move for improving the result. We will understand that we should reposition the experimental plan according to the dashed arrow. Zooming out and picturing what we have done on a map, we can see that we have only been exploiting a very small part of the entire experimental space. The true relationship between pH and volume is represented by the Contour Plot pictured below.
The amount of new content can be equated to the level of risk in the design or process. Product validation testing and prototype production runs are effective, but costly and in many cases problems are detected late in the development process. Engineers must use various analysis tools and statistical methods to reduce risk in a design or process. They must evaluate every change and how it could affect the process output. If you have multiple changes occurring at one time you could be multiplying your risk. So what can be done to predict how a set of changes will likely affect the process output?
Evaluating the Response

If you have a treatment group and a control group then, in this case, you probably only have one factor with two levels. So, if you used a 2-level full factorial design during the refinement and iteration stage, you only need to add the axial points and replicated center points to achieve an RSM design. Some DOE designs lend themselves to achieving the goals of more than 1 stage at a time, such as screening and optimization. We would have missed out acquiring the optimal temperature and time settings based on our previous OFAT experiments. These four points can be optimally supplemented by a couple of points representing the variation in the interior part of the experimental design. Next, we evaluate what will happen when we fix the volume at 550 ml (the optimal level) and start to change the second factor.
Because one component of DoE is the settings of factors, performing an experimental runs are applicable here. With DoE, factors are identified, responses are interpreted, and waste is eliminated or changed. Replication is the basic issue behind every method we will use in order to get a handle on how precise our estimates are at the end. We always want to estimate or control the uncertainty in our results. Another way we can achieve short confidence intervals is by reducing the error variance itself. However, when that isn't possible, we can reduce the error in our estimate of the mean by increasing n.
Research on surrogate model of dam numerical simulation with multiple outputs based on adaptive sampling ... - Nature.com
Research on surrogate model of dam numerical simulation with multiple outputs based on adaptive sampling ....
Posted: Mon, 24 Jul 2023 07:00:00 GMT [source]
Experiments are likely to be carried out via trial and error or one-factor-at-a-time (OFAT) method. We can see three main reasons that DOE Is a better approach to experiment design than the COST approach. In this way, DOE allows you to construct a carefully prepared set of representative experiments, in which all relevant factors are varied simultaneously.
These relationships or “interactions” often underpin complex and non-intuitive trends in the data which, in turn, hold key insight into the underlying biological complexity of a system or process. One-factor-at-a-time (OFAT) methods are incredibly common in biological research. One component (factor) is picked at a time and its values (levels) are varied, keeping all other known components constant.
This technique increases the experiment’s precision by controlling for block variation, allowing for a more accurate assessment of the treatment effects. Blocking showcases each experiment’s meticulous design and thoughtful consideration, highlighting the beauty in organizing complex data into understandable and meaningful patterns. Randomization is assigning subjects or experimental units to different groups in a study purely by chance. This critical process ensures that each group is comparable and extraneous variables do not bias the results. By eliminating potential bias, randomization safeguards the truthfulness of the experimental outcomes, making the findings generalizable and credible.
Doing a traditional DOE was not practical, so leadership decided to use conjoint analysis to help them design the optimal web page. DOE lets you balance trade-offs, such as what conditions produce the most cost-effective way to achieve the highest yield of strawberries. It’s a bit like trying to analyze the perfect cup of tea by ignoring the temperature of the water, brew time, and blend, and instead just focusing on whether you add the milk first or second.
It involves determining the relationship between input factors affecting a process and the output of that process. It helps to manage process inputs in order to optimize the output. Some knowledge of statistical tools and experimental planning is required to fully understand DOE methodology. While there are several software programs available for DOE analysis, to properly apply DOE you need to possess an understanding of basic statistical concepts. Blocking involves grouping similar experimental units and randomizing treatments within these blocks.
No comments:
Post a Comment